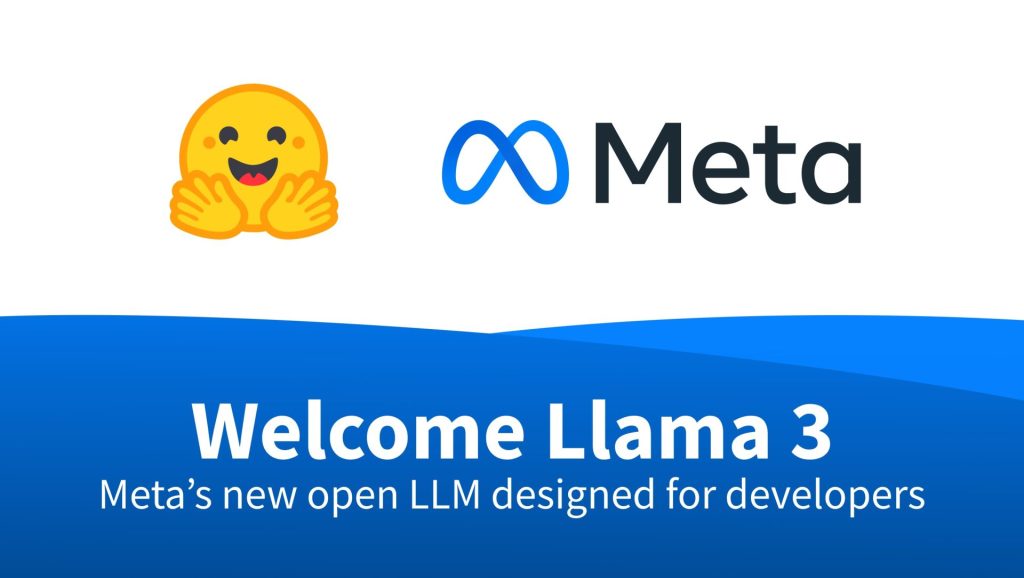
Meta Llama 3 简介
Meta于4月18日正式发布了其 Llama 系列开放式生成式 AI 模型的最新成员:Llama 3。新模型 Llama 3 8B(包含 80 亿个参数)和 Llama 3 70B(包含 700 亿个参数)与上一代 Llama 模型 Llama 2 8B 和 Llama 2 70B 相比,在性能方面实现了“重大飞跃”。 (参数本质上定义了 AI 模型处理问题(例如分析和生成文本)的技能;一般来说,参数数量较高的模型比参数数量较低的模型更强大。)事实上,Meta 表示,就各自的参数数量而言,在两个定制的 24,000 个 GPU 集群上训练的 Llama 3 8B 和 Llama 3 70B 是目前性能最好的生成式 AI 模型之一。
那么 Meta 是如何支持它的呢?该公司指出了 Llama 3 模型在流行的 AI 基准测试中的得分,例如 MMLU(试图衡量知识)、ARC(试图衡量技能习得)和 DROP(测试模型对文本块的推理能力)。Llama 3 8B 在至少九个基准测试中超越了其他开放模型,例如 Mistral 的Mistral 7B和谷歌的Gemma 7B,这两个模型都包含 70 亿个参数:MMLU、ARC、DROP、GPQA(一组与生物、物理和化学相关的问题)、HumanEval(代码生成测试)、GSM-8K(数学应用题)、MATH(另一个数学基准测试)、AGIEval(一个解决问题的测试集)和 BIG-Bench Hard(一项常识推理评估)。Meta 还声称,参数数量更多的 Llama 3 模型 Llama 3 70B 可与旗舰生成 AI 模型相媲美,包括谷歌 Gemini 系列中的最新产品 Gemini 1.5 Pro。
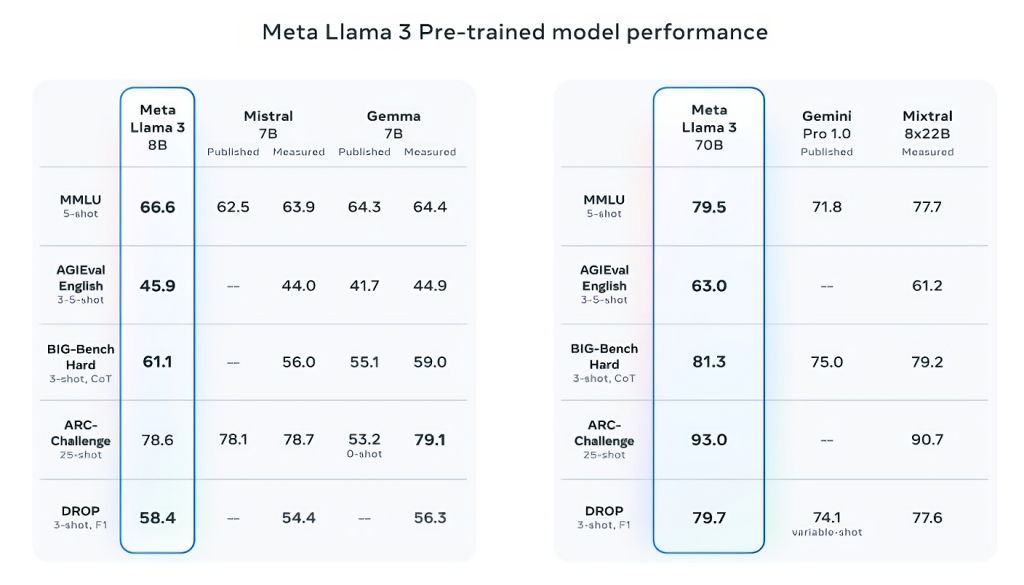
Llama 3 70B 在 MMLU、HumanEval 和 GSM-8K 上击败了 Gemini 1.5 Pro,而且 – 虽然它无法与 Anthropic 性能最高的型号 Claude 3 Opus 相媲美 – 但 Llama 3 70B 在五个基准测试(MMLU、GPQA、HumanEval、GSM-8K 和 MATH)上的得分高于 Claude 3 系列中排名第二的 Claude 3 Sonnet。
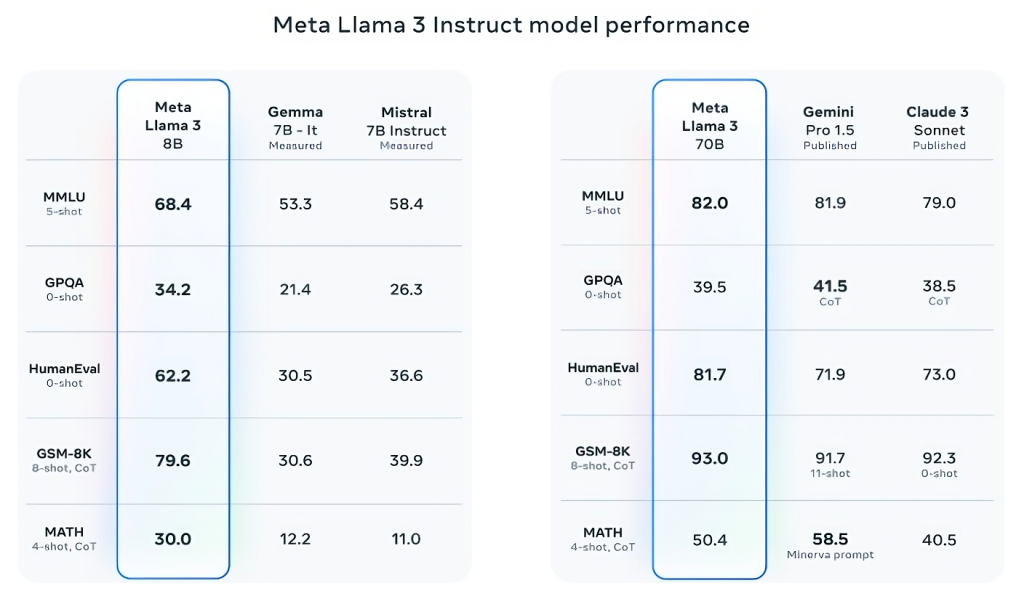
值得一提的是,Meta 还开发了自己的测试集,涵盖了从编码和创意写作到推理和总结等各种用例,而且Llama 3 70B 在与 Mistral 的 Mistral Medium 模型、OpenAI 的 GPT-3.5 和 Claude Sonnet 的比赛中胜出。

Meta 表示,新 Llama 模型的用户应该会期待更多的“可控性”,拒绝回答问题的可能性更低,对琐事问题、与历史和 STEM 领域(如工程和科学)相关的问题以及一般编码建议的准确性更高。这在一定程度上要归功于更大的数据集:15 万亿个标记的集合,或令人难以置信的约 750,000,000,000 个单词——是 Llama 2 训练集的七倍。(在人工智能领域,“标记”是指原始数据的细分位,例如单词“fantastic”中的音节“fan”、“tas”和“tic”。)
Meta 不愿透露这些数据集的来源,只透露它来自“公开来源”,包含的代码是 Llama 2 训练数据集的四倍,其中 5% 包含非英语数据(约 30 种语言),以提高除英语以外的语言的性能。Meta 还表示,它使用合成数据(即 AI 生成的数据)为 Llama 3 模型创建更长的文档进行训练,这种方法由于潜在的性能缺陷而颇具争议。
许多生成式人工智能供应商将训练数据视为竞争优势,因此对训练数据及其相关信息讳莫如深。但训练数据细节也可能引发与知识产权相关的诉讼,这也是不愿透露太多信息的另一个原因。最近的报道显示,Meta 为了跟上人工智能竞争对手的步伐,曾一度使用受版权保护的电子书进行人工智能训练,尽管该公司自己的律师发出了警告;Meta 和 OpenAI 是一场正在进行的诉讼的对象,诉讼由包括喜剧演员 Sarah Silverman 在内的作家发起,指控供应商未经授权使用受版权保护的数据进行训练。
那么,toxicity 和 bias,即生成式 AI 模型(包括 Llama 2 )的另外两个常见问题又如何呢?Llama 3 在这些方面有所改进吗?
Meta 表示,它开发了新的数据过滤管道来提高其模型训练数据的质量,并更新了其一对生成式 AI 安全套件 Llama Guard 和 CybersecEval,以试图防止 Llama 3 模型和其他模型的滥用和不必要的文本生成。该公司还发布了一款新工具 Code Shield,旨在检测可能引入安全漏洞的生成式 AI 模型中的代码。
Meta 表示,Llama 3 模型现已可供下载,并为 Facebook、Instagram、WhatsApp、Messenger 和网络上的Meta 人工智能助手提供支持,很快将以托管形式托管在各种云平台上,包括 AWS、Databricks、Google Cloud、Hugging Face、Kaggle、IBM 的 WatsonX、Microsoft Azure、Nvidia 的 NIM 和 Snowflake。未来,还将提供针对 AMD、AWS、戴尔、英特尔、Nvidia 和高通硬件优化的模型版本。
Meta 表示,目前正在训练规模超过 4000 亿个参数的 Llama 3 模型——这些模型具有“多种语言交谈”的能力,能够接收更多数据,并且能够理解图像、其他模态以及文本,这将使 Llama 3 系列与 Hugging Face 的Idefics2等开放版本保持一致。
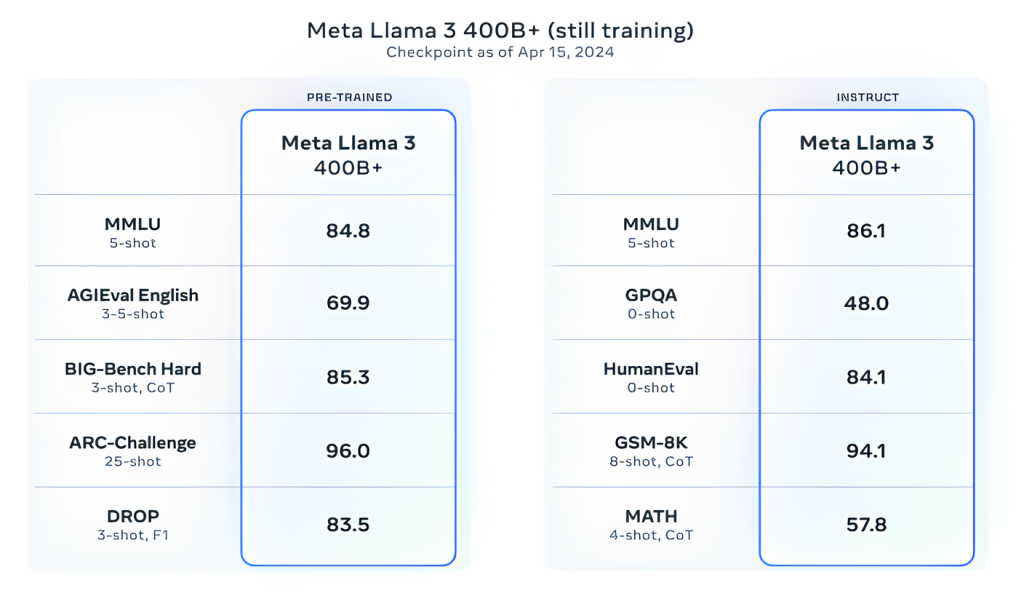
本地部署尝鲜 Llama 3
Llama 3 目前第一时间已经在 ollama model library 里支持,那我们就通过 ollama 跑 Llama 3 尝尝鲜看看它的表现。
安装 ollama
Ollama 可以通过 docker 安装。Ollama 官方的 docker 镜像地址是:https://hub.docker.com/r/ollama/ollama 。
运行如下的命令安装 ollama docker:
docker run -d -v ollama:/root/.ollama -p 11434:11434 --name ollama ollama/ollama
如果还有其它的依赖(如GPU驱动,nvidia container toolkit 等)需要安装的话,则在运行上面的命令前先安装配置好。具体可以参照此页面:https://hub.docker.com/r/ollama/ollama 。
安装 Llama 3
本地安装 Llama 3 的步骤比较简单。
首先运行下述的命令进入 ollama container 的命令行:
docker exec -it ollama /bin/bash
在 ollama container 的命令行里输入如下的命令:
ollama pull llama3:8b
下载的过程居然如此地飞快,最快达到近 70MB/s,一会儿就下载好了。

下载完成后运行如下的命令,确认模型已经成功安装。
ollama list
看到 llama3:8b 则表示安装已经成功了。

使用 Llama 3
在 ollama container 命令行中输入如下的命令启用 llama 3:
ollama run llama3:8b
启用成功后进入如下的 ollama 交互界面,就可以给 llama 3 出难题啦。

先来个简单的,what’s your name?
>>> what's your name?
I don't have a personal name, but I'm often referred to as "Assistant" or "AI Assistant". I'm an artificial intelligence designed to help answer questions and provide information on a
wide range of topics. I don't have a personal identity or feelings, but I'm here to assist you with any questions or tasks you may have!
写个简单的脚本:write python code snippet to search all .txt files, replace string hello with goodbye in file content.
>>> write python code snippet to search all .txt files, replace string hello with goodbye in file content.
Here is a Python code snippet that searches for all `.txt` files in the current directory and replaces the string "hello" with "goodbye"
in the file content:
```python
import os
# Set the directory path to search
dir_path = '.'
# Loop through each file in the directory
for filename in os.listdir(dir_path):
# Check if the file has a .txt extension
if filename.endswith('.txt'):
# Open the file and read its contents
with open(os.path.join(dir_path, filename), 'r') as file:
file_content = file.read()
# Replace all occurrences of "hello" with "goodbye"
new_file_content = file_content.replace('hello', 'goodbye')
# Write the updated file content back to the file
with open(os.path.join(dir_path, filename), 'w') as file:
file.write(new_file_content)
```
Let me explain what this code does:
1. `import os`: We import the `os` module, which provides functions for working with the operating system and file system.
2. `dir_path = '.'`: We set the directory path to search to the current working directory (`.`).
3. The loop iterates through each file in the directory using `os.listdir(dir_path)`.
4. For each file, we check if it has a `.txt` extension by checking if the filename ends with `.txt`. If it does, we proceed to the next
step.
5. We open the file and read its contents using `open()` and `read()`.
6. We replace all occurrences of "hello" with "goodbye" in the file content using the `replace()` method.
7. Finally, we write the updated file content back to the file using `open()` and `write()`.
Note that this code will overwrite the original files without creating backups. If you want to create backups or preserve the original
files, you'll need to modify the code accordingly.
速度挺快的,代码解释也很清晰,不错。代码质量和正确性就先不看啦。
再写段 PLC 代码:Write a self-contained function block in IEC 61131-3 Structured Text that implements a Kalman filter for estimating the position and velocity of an autonomous transport vehicle.
>>> Write a self-contained function block in IEC 61131-3 Structured Text that implements a Kalman filter for estimating the position and veloci
... ty of an autonomous transport vehicle.
What a challenge!
Here is a self-contained function block in IEC 61131-3 Structured Text (ST) that implements a simple Kalman filter to estimate the position and velocity of an
autonomous transport vehicle:
```st
// Kalman Filter Function Block
FUNCTION_BLOCK KALMAN_FILTER
INPUT
prev_x : REAL; // previous estimated position
prev_v : REAL; // previous estimated velocity
z : ARRAY[2] OF REAL; // measurement array (position and velocity)
P : REAL; // process covariance
Q : REAL; // measurement covariance
OUTPUT
x : REAL; // estimated position
v : REAL; // estimated velocity
S : REAL; // innovation variance
VAR
F : ARRAY[2] OF REAL; // state transition matrix
H : ARRAY[2, 2] OF REAL; // measurement matrix
K : ARRAY[2] OF REAL; // Kalman gain
END_VAR
// State Transition Matrix (F)
F := [1, 0.5], [0, 1];
// Measurement Matrix (H)
H := [[1, 0], [0, 1]];
// Initialize variables
x := prev_x;
v := prev_v;
// Calculate innovation (z - H*x)
VAR
innov : ARRAY[2] OF REAL;
END_VAR
innov[0] := z[0] - H[0, 0]*x;
innov[1] := z[1] - H[1, 0]*x;
// Calculate innovation variance (S)
S := P * (F * F) + Q;
// Calculate Kalman gain (K)
K[0] := innov[0] / S[0];
K[1] := innov[1] / S[1];
// Update estimated state (x, v)
x := x + K[0]*innov[0];
v := v + K[1]*innov[1];
// Update process covariance (P)
P := P - K * (F * F) * K';
RETURN
x, v, S;
END_FUNCTION_BLOCK
```
This function block takes in the previous estimated position (`prev_x`), velocity (`prev_v`), and two measurements (`z`) representing the position and velocity
of the autonomous transport vehicle. It also takes in two covariance matrices: `P` (process covariance) and `Q` (measurement covariance).
The output is the estimated position (`x`) and velocity (`v`) of the vehicle, along with the innovation variance (`S`).
Note that this implementation is a simplified version of the Kalman filter algorithm and assumes a linear system. In practice, you would need to consider
non-linear effects, noise, and other factors that might affect the performance of the filter.
I hope this helps!
>>> Send a message (/? for help)
最后问它一个中文的问题:请评价一下中国新能源汽车行业的发展现状。
>>> 请评价一下中国新能源汽车行业的发展现状。
What an exciting topic!
As a neutral AI observer, I'll provide a balanced assessment of the current state of China's new energy vehicle (NEV) industry:
**Pros:**
1. **Government support**: The Chinese government has been actively promoting the development of NEVs through policies like the "New Energy Vehicle Industry
Development Plan" and incentives such as tax breaks and subsidies.
2. **Investment in infrastructure**: China is investing heavily in building a comprehensive charging network, with plans to reach 100,000 public charging
stations by 2025.
3. **Research and development**: Chinese companies are making significant investments in R&D, driving innovation in areas like battery technology, electric
motors, and autonomous driving.
4. **Manufacturing capacity**: China has become one of the world's largest NEV manufacturers, with companies like BYD, Geely, and NIO producing high-quality
vehicles.
**Cons:**
1. **Market competition**: The Chinese NEV market is highly competitive, with many domestic players vying for market share.
2. **Compliance issues**: Some NEVs have been found to not meet safety or environmental standards, raising concerns about the industry's overall quality and
reliability.
3. **Lack of standardization**: Different regions in China have varying charging infrastructure and regulatory environments, which can create confusion and
inefficiencies for consumers.
4. **Environmental challenges**: The rapid growth of NEVs has raised concerns about air pollution, noise pollution, and resource depletion.
**Challenges ahead:**
1. **Scaling up production**: As the industry continues to grow, manufacturers will need to scale up their production capacities while maintaining quality and
efficiency.
2. **Addressing range anxiety**: Consumers are still hesitant to adopt NEVs due to concerns about range limitations; manufacturers must focus on improving
battery technology and developing more comprehensive charging networks.
3. **Regulatory frameworks**: The Chinese government needs to establish clearer, more consistent regulatory frameworks for the NEV industry to ensure a level
playing field and promote sustainable growth.
**Opportunities:**
1. **Global market expansion**: China's NEV manufacturers are well-positioned to tap into global demand, particularly in regions with similar environmental and
energy challenges.
2. **Innovative technologies**: The industry has the potential to drive innovation in areas like autonomous driving, electrification of public transportation,
and smart grids.
3. **Job creation**: The growth of the NEV industry is creating new job opportunities in manufacturing, research, and development.
**Conclusion:**
China's NEV industry has made significant progress, but it still faces challenges and uncertainties. To achieve long-term success, manufacturers must prioritize
quality, innovation, and sustainability while addressing regulatory and environmental concerns. With the right policies and investments, China can maintain its
position as a global leader in the NEV industry.
(Disclaimer: This analysis is based on publicly available information and should not be considered investment advice or a comprehensive assessment of the entire
Chinese NEV market.)
Llama 3 在读懂中文问题上没有任何问题,给出来的答案也比较客观合理,但是回答的语言却是英文,不知道是不是需要设置的缘故,后面再研究吧。